Current 3D AD&S
We begin our investigation by evaluating if current 3D AD&S methods are actually better than the SoTA 2D methods when applied on 3D data. Surprisingly, PatchCore, which does not use 3D information, outperforms all previous methods.
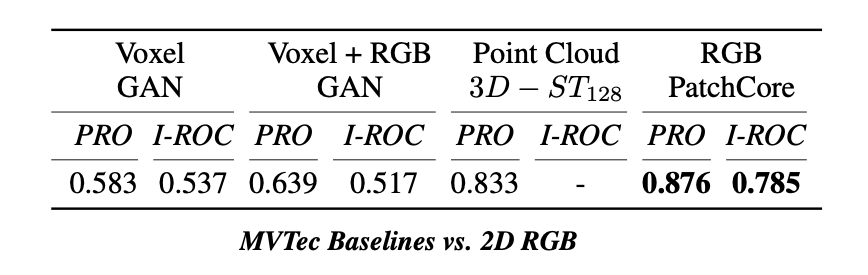
Despite significant advances in image anomaly detection and segmentation, few methods use 3D information. We utilize a recently introduced 3D anomaly detection dataset to evaluate whether or not using 3D information is a lost opportunity. First, we present a surprising finding: standard color-only methods outperform all current methods that are explicitly designed to exploit 3D information. This is counter-intuitive as even a simple inspection of the dataset shows that color-only methods are insufficient for images containing geometric anomalies. This motivates the question: how can anomaly detection methods effectively use 3D information? We investigate a range of shape representations including hand-crafted and deep-learning-based; we demonstrate that rotation invariance plays the leading role in the performance. We uncover a simple 3D-only method that beats all recent approaches while not using deep learning, external pre-training datasets, or color information. As the 3D-only method cannot detect color and texture anomalies, we combine it with color-based features, significantly outperforming previous state-of-the-art. Our method, dubbed BTF (Back to the Feature) achieves pixel-wise ROCAUC: 99.3% and PRO: 96.4% on MVTec 3D-AD.
We begin our investigation by evaluating if current 3D AD&S methods are actually better than the SoTA 2D methods when applied on 3D data. Surprisingly, PatchCore, which does not use 3D information, outperforms all previous methods.
We also investigate whether 3D information is needed
Our investigation examines a large range of approaches including handcrafted descriptors and pre-trained deep features for both depth and 3D approaches. Our surprising result is that a classical 3D handcrafted point cloud descriptor outperforms all other 3D methods - including all previously presented baselines as well as a concurrent deep-learning-based point cloud approach.
@inproceedings{horwitz2023back,
title={Back to the feature: classical 3d features are (almost) all you need for 3d anomaly detection},
author={Horwitz, Eliahu and Hoshen, Yedid},
booktitle={Proceedings of the IEEE/CVF Conference on Computer Vision and Pattern Recognition},
pages={2968--2977},
year={2023}
}